How we're building our Matching Science to overcome the "black box problem".
Team update: A lot has happened since this blog - all great things! We’re now in the United States and our new product launched in November 2021, helping teams in fast-growing organisations find and hire their best-fit junior and mid-level talent in Sales, Marketing, Operations, and Customer Success. Try it here for free. This means some of our articles before this date may have product shots that look a little different. That’s all from us, enjoy the blog.
We’ve all heard about “black box” AI systems that make decisions, and no one knows why. GDPR encodes a “right to explanation”, which is technically challenging to meet in many AI use cases. Our Matching Science's accuracy and rigour is central to our product and mission. We believe that providing clear reasoning behind our Matching to both candidates and company users is a core part of the user experience, not a compliance exercise.
Our solution is to build explainability into our matching from the ground up. We do this via a Knowledge Graph that functions as the spine of our matching; enabling us to control the way each data input influences a matching outcome (such as the decision to shortlist a candidate).
Our knowledge graph acquires and integrates information, structuring the data in a way that's easily adaptable over time. It provides us with a programmatic way to model our body of knowledge about different role types with the help of subject-matter experts, data interlinking and inputs from our users. It enables us to embed our current beliefs on concepts relating to work and jobfit (like skill and capabilities) at different levels of granularity.
When we think of a job, there are a number of skill levels varying in importance required to do the job. However, each company operates within a different context, influenced by several different internal and external factors that may change or shift the necessary skill level required or its importance. How can we best capture what we know generally, but also the intricacies the specific role requires?
The knowledge graph leverages information from O*Net to capture insights for every job code. O*Net is a contemporary, interactive skills-based database that provides a common language to describe worker skills, attributes and work activities associated with occupations. O*Net provides us with an evidence based foundation that when encapsulated by the knowledge graph, can provide the modelling framework to reason and adjust for the specifics of an individual role we’re working on.
As O*Net’s information encapsulates a broad knowledge of what a role requires, it can also help us understand other roles a candidate may be good at, by simply asking the knowledge graph what other roles best utilise the candidate’s skills.
All mathematical graphs consist of two different elements; nodes and edges. In our knowledge graph, the nodes represent elements of the matching world we are interested in, such as transferable skills, knowledge, and skill sets. The edges between nodes represent relationships between these elements, such as the level of knowledge this capability requires, or the importance of this skill in a skill set.
We can then use the knowledge graph to provide a baseline set of beliefs about a role, telling us what skills are needed, and by proxy, what capabilities are important to help us better understand the role we’re matching to. Our matching science then adjusts these beliefs as we learn more about what's unique to the role in a specific company's context.
So, what does applying the knowledge graph to our matching science look like for a real role?
Say you work for a fintech company and are looking for a data analyst; we would look at what skill sets exist in O*Net already. For instance, a good starting point would be to look at the skill set for a Financial Analyst.
As part of our job analysis process, we dig into your requirements to find out more about your specific role.
In this example, your team's data analyst will need to interact with and present solutions to clients. To cater to this, we can build upon the skill set by adding the capability of developing client-focused solutions which then tells us more about the range of the skill you need.
We can then use this information to determine the job application's design and the assessment criteria to find candidates who are the right match for your specific role. You’ll receive all of this information in a Match Profile to review and approve before the role goes live.
Here’s a quick look at the Match Profile for the example Data Analyst role:
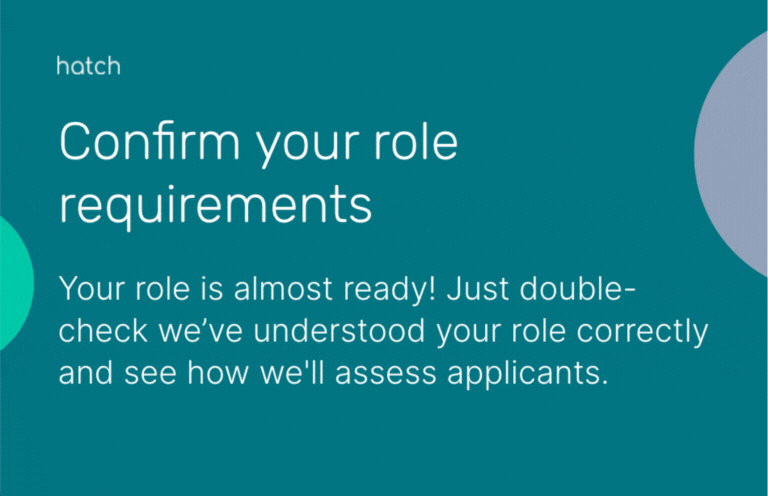
We’re continuing to map out how this information impacts what we assess and use our learnings from shortlists delivered as an input into a feedback loop for our Matching Science. The knowledge graph is how we have encapsulated our current beliefs on the concepts involved in job fit. As we learn and update our beliefs over time, we can update the knowledge graph to reflect our new beliefs.